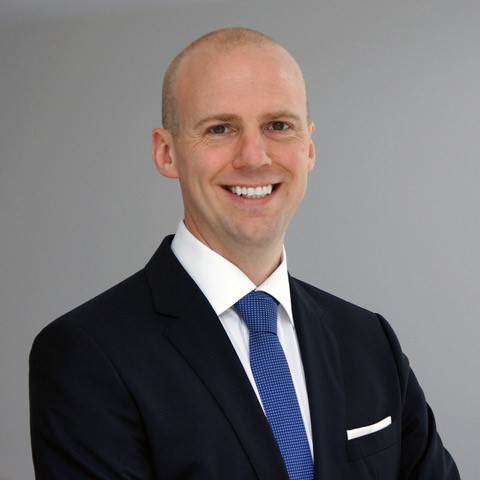
A recent Forrester surveyof 433 procurement, supply chain, and finance leaders found that more than half (55 percent) plan to invest in Artificial Intelligence within the next two years. However, 59 percent voiced concerns about poor data quality limiting the effectiveness of A.I. insights and decision-making. There’s clearly a lot of work ahead, but the obstacles are far from insurmountable.
Here are four areas where A.I. could transform supply chains.
Stock redistribution
Many of the areas we’ve already discussed could merge to transform the way stock levels and optimal distribution is currently decided upon. Machine learning coupled with more IoT sensors will provide an increasingly accurate real-time view of precisely where every item of stock is, whether it’s an outgoing order in transit, a return or repair, sitting in a warehouse, or on the shelves of a store.
Analysis of likely demand and a complete picture of the associated costs of storing stock in different locations can be combined by A.I. to reroute and distribute stock in the most efficient way. There are huge financial benefits for companies that can maintain the right stock levels to fulfil demand and optimize reverse logistics.
As the success stories around A.I begin to roll in and confidence grows, more services and products will become available, lowering the barriers to entry for interested organizations keen to transform their supply chains.
Automated problem resolution
With visibility and control enabling supply chain orchestration you can use a control tower to configure acceptable parameters for any individual order in terms of cost, delivery time, and other constraints. Instead of someone having to make a decision and reroute an order in the event of a problem, A.I. can automate the process, detecting when an order is going to veer outside one of its set parameters and making real-time changes to correct. It may even make proactive suggestions when a cheaper or faster route for an order presents itself.
Automated issue resolution could be a huge time and cost saving. Just like chatbots or automated quality inspection, it also has the potential to free up human expertise. And in borderline cases or unusual circumstances, it can flag problems for further investigation or wait for a final decision to be made by an experienced employee.
Automate repetitive manual tasks
Machine learning has proven adept at pattern recognition and there is major potential to leverage it for visual inspections. Quality control for products, outbound and inbound package inspections, and damage identification are all tasks that A.I. could assume with a higher degree of consistency and speed than manual workers.
Beyond the initial investment, employing automated systems will reduce long term costs, allowing organizations to grow while maintaining a steady or even reduced headcount. Anything that falls outside the scope of machine learning models can be flagged for human inspection.
Chatbots for customers and partners
There are more than 265 billion customer service calls every year at a cost of $1.3 trillion for businesses, according to IBM, yet chatbots could be employed to answer up to 80 percent of routine questions.
Customers demand greater transparency into the order life cycle nowadays and they use a much wider array of different channels to communicate with suppliers. Chatbots can boost response times for generic queries and free up agents to deal with more complex problems.
Employing A.I. in this way could save companies a great deal of money and make it easier to provide 24/7 customer support.
Every conversation is a learning opportunity and customer service calls with human agents can also serve as instructional data enabling chatbots to improve rapidly. Patterns around how the chatbots handle customer service calls will gradually start to emerge enabling preventative measures to be taken at the source of the concern. Customers will be unable to distinguish between A.I. and human agents in many circumstances and there’s scope for a seamless hand-off to a human when the chatbot is unable to satisfy a customer.
There are several important applications for machine learning and A.I. in the supply chain that have the potential to drive tangible gains. Many organizations are weighing their options or looking to invest but ensuring the right focus for the best return requires some careful research and planning.
About the Author
Peter Nilsson is the Vice President of Strategic Initiatives for MP Objects, a leading multi-enterprise cloud platform for digital order management and supply chain orchestration. He was previously Director, Solutions Strategy for GT Nexus and prior to that he worked for Capgemini BPO. Contact him at peter.nilsson@mp-objects.com.